•
Author: Christophe Dessimoz •
∞
The recent publication of our paper “Current methods for automated filtering of multiple sequence alignments frequently worsen single-gene phylogenetic inference” in Systematic Biology is the conclusion of 5 years of work, most of which was spent in peer-review. I will write a separate post on the issue of pre- vs. post-publication in a later post (update: available here). For now, I summarise our main results, try to provide an intuition for them, and give the story behind the paper.
Does automatic alignment filtering lead to better trees?
One major use of multiple sequence alignments is for tree inference. Because aligners make mistakes, many practitioners like to mask the uncertain parts of the alignment. This is done by hand or using automated tools such as Gblocks, TrimAl, or Guidance.
The aim of our study was to compare different automated filtering methods and assess under which conditions filtering might be beneficial. We compared ten different approaches on several datasets covering hundreds of genomes across different domains of life—(including nearly all of the Ensembl database) as well as simulated data. We used several criteria to assess the impact of filtering on tree inference (comparing the congruence of resulting trees with undisputed species trees and counting the number of gene duplications implied). We sliced the data in many different ways (sequence length, divergence, “gappyness”).
The more we filter alignments, the worse trees become.
In all datasets, tests, and conditions we tried, we could hardly find any situation in which filtering methods lead to better trees; in many instances, the trees got worse:
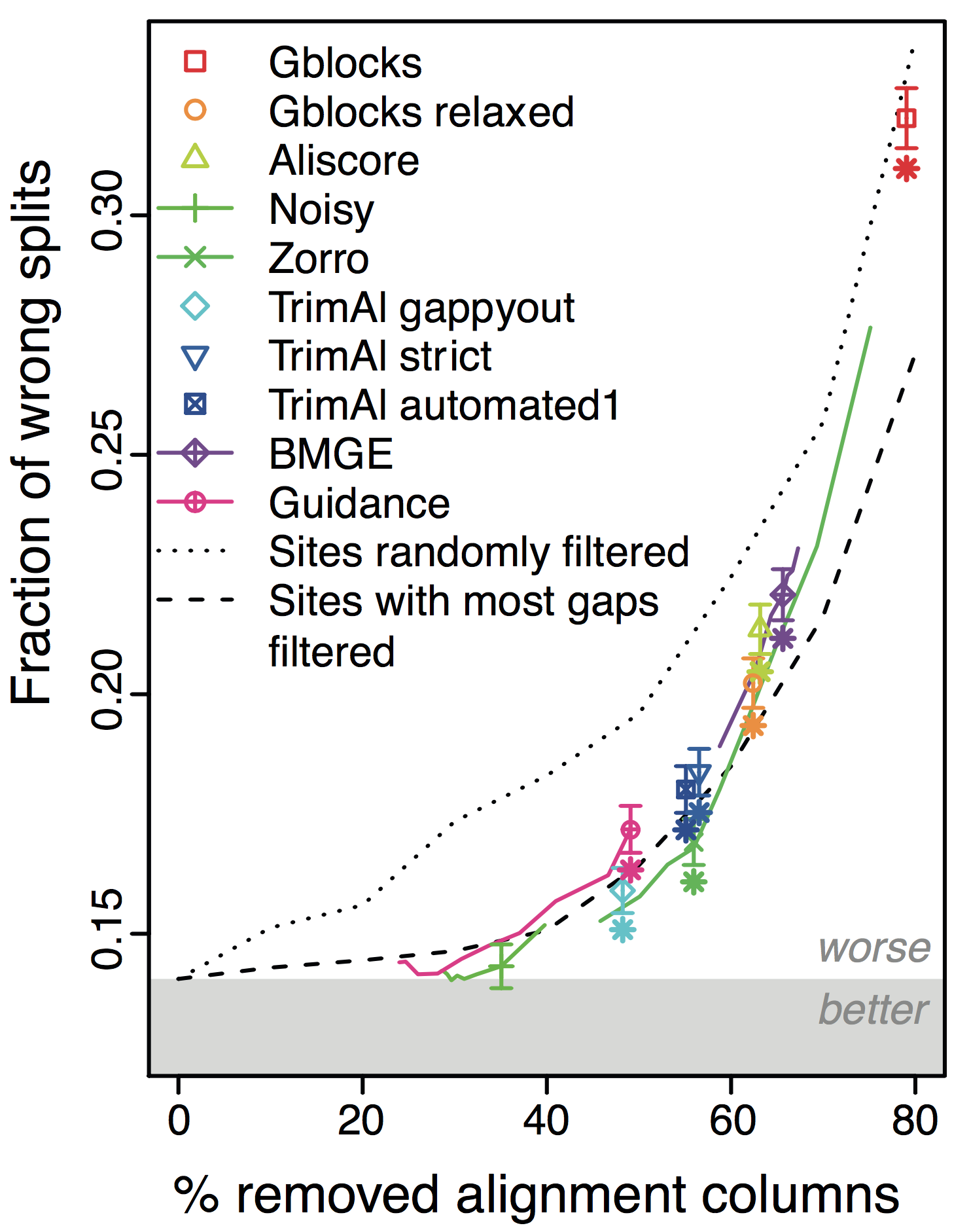
Overall, the more alignments get filtered (x-axis in figure), the worse the trees become! This holds across different datasets and filtering methods. Furthermore, under default parameters, most methods filter far too many columns.
The results were rather unexpected, and potentially controversial, so we went to great lengths to ensure that they were not spurious. This included many control analyses and replication of the results on different datasets, and using different criteria of tree quality. We also used simulated data, for which the correct tree is known with certainty.
What could explain this surprising result?
It appears that tree inference is more robust to alignment errors than we
might think. One hypothesis for this might be that while alignment
errors introduce mostly random (unbiased) noise, correct columns (or partly
correct ones) contain crucial phylogenetic signal that can help discriminate
between the true and alternative topologies.
But why could this be the case? We are not sure, but here is an idea: aligners
tend to have most difficulty with highly distant sequences, because there are
many evolutionary scenarios that could have resulted in the same sequences. At
the limit, if the distance is very large (e.g. sites have undergone multiple
substitions on average), all alignments become equally likely, and it becomes
impossible to align the sequences. Also, the variance of the distance estimate
explodes. But relative to this enormous variance,
the bias introduced by alignment errors becomes negligible.
I stress that we don’t prove this in the paper and this is merely a conjecture
(some might call this posthoc rationalisation).
So is filtering an inherently bad idea?
Although alignment filtering does not improve tree accuracy, we can’t say that
it is inherently a bad idea. Moderate amounts of filtering did not seem to have much
impact—positive or negative—but can save some computation time.
Also, if we consider the accuracy of the alignment themselves, which we did in
simulations (such that we know the true alignment), filtering does decrease
the proportion of erroneous sites in the aligments (though, of course, these
alignments get shorter!). Thus for
applications more sensitive to alignment errors than tree inference, such as detection of
sites under positive selection, it is conceivable that filtering might, in
some circumstances, help. However, the literature on the topic is rather
ambivalent (see here, here, here, and here).
Why it took us so long: a brief chronology of the project
The project started in summer 2010 as a 3-week rotation project by Ge Tan, who was a talented MSc student at ETH Zurich at the time (he is now a PhD student at Imperial College London, in Boris Lenhard’s group). The project took a few months more than originally foreseen to complete, but early-on the results were already apparent. In his report, Ge concluded:
“In summary, the filtering methods do not help much in most cases.”
After a few follow-up analyses to complete the study, we submitted a first manuscript to MBE in Autumn 2011. This first submission was rejected after peer-review due to insufficient controls (e.g. lack of DNA alignments, no control for sequence length, proportion of gaps, etc.). The editor stated:
“Because the work is premature to reach the conclusion, I cannot help rejecting the paper at this stage”.
Meanwhile, having just moved to EMBL-EBI near Cambridge UK, I gave a seminar on the work. Puzzled by my conclusions, Matthieu Muffato and Javier Herrero from the Ensembl Compara team set out to replicate our results on the Ensembl Compara pipeline. They saw the same systematic worsening of their trees after alignment filtering.
We joined forces and combined our results in a revised manuscript, alongside additional controls requested by the reviewers from our original submission. The additional controls necessitated several additional months of computations but all confirmed our initial observations. We resubmitted the manuscript to MBE in late 2012 alongside a 10-page cover letter detailing the improvements.
Once again, the paper was rejected. Basically, the editor and one referee did not believe in the conclusions and no amount of controls were going to convince them of the contrary. We appealed. The editor-in-chief rebutted the appeal but now the reason was rather different:
“[Members of the Board of Editors] were not convinced that the finding that the automated filtering of multiple alignment does not improve the phylogenetic inference on average for a single-gene data set was sufficiently high impact for MBE.”
We moved on and submitted our work to Systematic Biology. Things worked out better there, but it nevertheless took another two years and three resubmissions—addressing a total of 147 major and minor points (total length of rebuttal letters: 43 pages)—before the work got accepted. Two of the four peer-reviewers went so far as to reanalyse our data as part of their report—one conceding that our results were correct and the other one holding out until the bitter end.
Why no preprint?
Some of the problem with this slow publication process could would have been
mitigated if we had submitted the paper as a preprint. In hindsight, it’s
obvious that we should have done so. Initially, however, I did not anticipate
that it would take so long. And with each resubmission, the paper was
strengthening so I thought during the whole time that it was just about to be accepted… Also, I surely
also fell for the Sunk Cost
Fallacy.
Other perils of long-term projects
I’ll finish with a few amusing anectodes highlighting the perils of papers requiring many cycles of resubmissions:
- More than once, we had to redo analyses with new filtering methods that got published after we started the project.
- At some point, one referee asked why we were using such an outdated version of TCoffee (went from version 5 to version 10 during the project!).
- The editor-in-chief of MBE changed, and alongside some of the editorial policy and manuscript format (the paper had to be restructured with the method section at the end).
Reference
Tan, G., Muffato, M., Ledergerber, C., Herrero, J., Goldman, N., Gil, M., & Dessimoz, C. (2015). Current Methods for Automated Filtering of Multiple Sequence Alignments Frequently Worsen Single-Gene Phylogenetic Inference Systematic Biology, 64 (5), 778-791 DOI: 10.1093/sysbio/syv033
If you enjoyed this post, you might want to check the other entries of our
series “story behind the paper”.
•
Author: Christophe Dessimoz •
∞
The new academic year brings a big change to our lab. I am moving to the
University of Lausanne, Switzerland, on a professorship
grant from the Swiss National Science Foundation. The
generous funding will enable us to expand our activities on computational
methods dealing with mixtures of phylogenetic
histories. Lausanne is a hub
for life sciences and bioinformatics so we will feel right at home there—indeed
we have already been collaborating with several
groups
there. I
join the Center for Integrative Genomics and the
Department of Ecology and Evolution. I also look
forward to reintegrating the Swiss Institute of Bioinformatics. At a personal
level, this marks a return to a region in which I grew up, after 16 years in
exile.
However, I keep a joint appointment at UCL, where part
of the lab remains. I’ll be flying back regularly and keep some of my
teaching
activities. UCL is a very special place—one which would be too hard for me to leave
entirely. For all the cynicism we hear about universities-as-businesses, the
overriding priority at UCL clearly remains on outstanding scholarship. My
departments (Genetics, Evolution, Environment and
Computer Science) are both highly collegial and
supportive. Compared to the previous institutions I have worked for, the
organisational culture at UCL is very much bottom-up. The pervasive chaos is
perceived as a shortcoming by some, but it’s actually a huge competitive
advantage—one that leaves ample room for initiative and flexibility. One
colleague once told me that I could build a nuclear reactor in my lab and no
one would ask a question—provided I secure the funding for it of course…
So how are we going to manage working in two different sites? Well, the
situation is not new. We have had a distributed lab for several years and have
developed a system for remote collaboration. Currently, we have lab members
primarily based in London, Zurich, Ghent, and Cambridge. Our weekly lab
meeting and monthly journal club are done via videoconference (with
GoToMeeting). I try to have at least fortnightly 1:1
meetings with all remote members. During the day, the lab stays in touch via
instant messaging (using HipChat). We have shared code
(git) and data (sshfs) repositories. We tend to write collaborative papers
using Google Docs (with Paperpile as reference
manager). Importantly, we have a lab retreat every four months where we meet
in person, reflect on our work, and
have
fun. We
supplement this with collaborative visits as needed. The system is not
perfect—please share your experience if you’ve found other good ways of
collaborating remotely—but overall it’s working quite well.